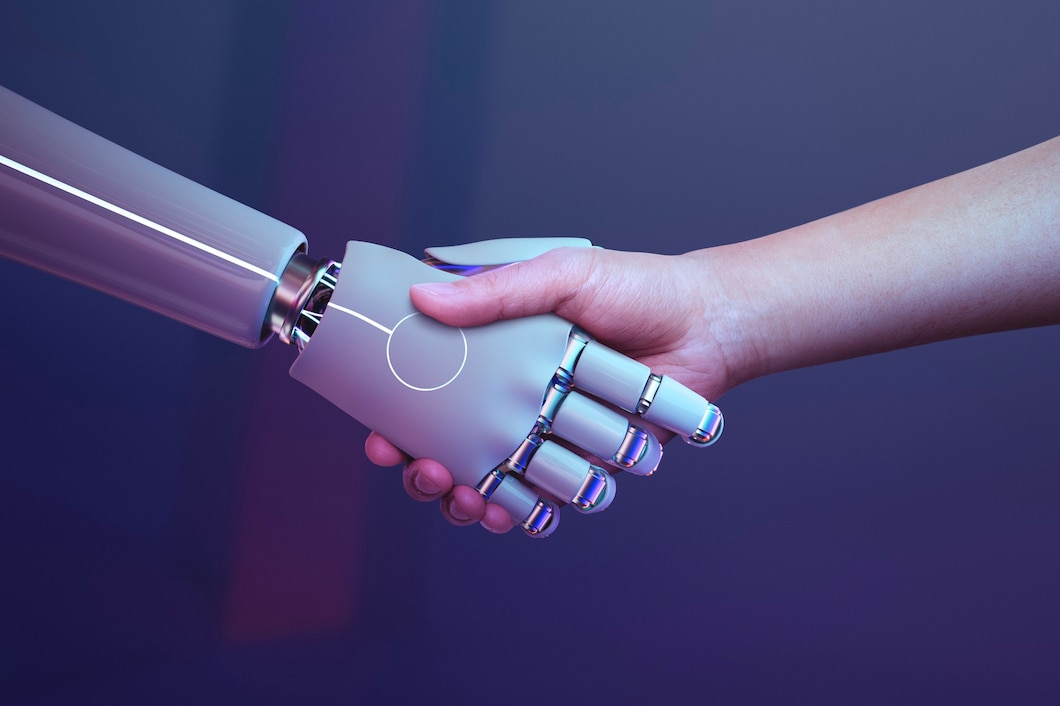
Artificial intelligence is designed to assist with decision-making when the data, parameters, and variables involved are beyond human comprehension. For the most part, AI systems make the right decisions given the constraints. However, AI notoriously fails in capturing or responding to intangible human factors that go into real-life decision-making — the ethical, moral, and other human considerations that guide the course of business, life, and society at large.
Consider the “trolley problem” — a hypothetical social scenario, formulated long before AI came into being, in which a decision has to be made whether to alter the route of an out-of-control streetcar heading towards a disaster zone. The decision that needs to be made — in a split second — is whether to switch from the original track where the streetcar may kill several people tied to the track, to an alternative track where, presumably, a single person would die.
While there are many other analogies that can be made about difficult decisions, the trolley problem is regarded to be the pinnacle exhibition of ethical and moral decision making. Can this be applied to AI systems to measure whether AI is ready for the real world, in which machines can think independently, and make the same ethical and moral decisions, that are justifiable, that humans would make?
Trolley problems in AI come in all shapes and sizes, and decisions don’t necessarily need to be so deadly — though the decisions AI renders could mean trouble for a business, individual, or even society at large. One of the co-authors of this article recently encountered his own AI “trolley moment,” during a stay in an Airbnb-rented house in upstate New Hampshire. Despite amazing preview pictures and positive reviews, the place was poorly maintained and a dump with condemned adjacent houses. The author was going to give the place a low one-star rating and a negative review, to warn others considering a stay.
However, on the second morning of the stay, the host of the house, a sweet and caring elderly woman, knocked on the door, inquiring if the author and his family were comfortable and if they had everything they needed. During the conversation, the host offered to pick up some fresh fruits from a nearby farmers market. She also said she doesn’t have a car, she would walk a mile to a friend’s place, who would then drive her to the market. She also described her hardships over the past two years, as rentals slumped due to Covid and that she is caring for someone sick full time
Upon learning this, the author elected not to post the negative review. While the initial decision — to write a negative review — was based on facts, the decision not to post the review was purely a subjective human decision. In this case, the trolley problem was concern for the welfare of the elderly homeowner superseding consideration for the comfort of other potential guests.
How would an AI program have handled this situation? Likely not as sympathetically for the homeowner. It would have delivered a fact-based decision without empathy for the human lives involved.
AI’s Mixed Record as Ultimate Decision-Maker
AI has progressed to compete with the best of the human brain in many areas, often with stunning accuracy, quality, and speed. But can AI introduce the more subjective experiences, feelings, and empathy that makes our world a better place to live and work, without cold, calculating judgment? Hopefully, but that remains to be seen. The bottom line is, AI is based on algorithms that responds to models and data, and often misses the big picture and most times can’t analyze the decision with reasoning behind it. It isn’t ready to assume human qualities that emphasize empathy, ethics, and morality.
AI may not be as advanced as many would like when it comes to looking at the total context of other real-world situations it encounters, and its decisions may be consequential. Consider these relatively recent incidents cited in news reports:
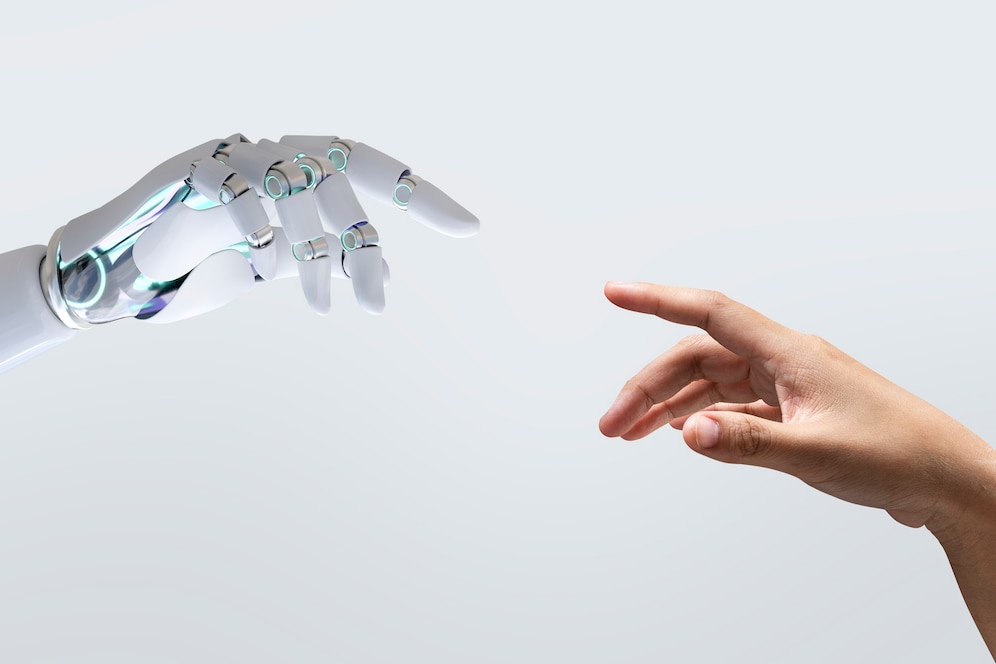
Driving while autonomous.
An Uber self-driving experiment was called off after the self-driving car killed a pedestrian in Tempe, Arizona. The victim was fatally struck by the Uber test vehicle while pushing a bicycle across a four-lane road away from a crosswalk. A human driver would have realized this and potentially stopped the vehicle. The vehicle had a backup driver aboard, but the driver was watching streaming video and therefore was distracted at the critical moment which could have potentially avoided the fatality. While human error was first blamed, the National Transportation Safety Board determined the AI failed to classify the jaywalking pedestrian as such, as the object was not near a crosswalk as expected under normal circumstances. This means the training and AI models were not properly implemented.
Recruiting bias.
Amazon built an AI-based tool to “out recruit” other tech firms in the tech brains arms race. The company trained their models to look for top talent in the resumes. However, the AI models were trained using tainted data collected over a 10-year period in which the vast majority of candidates were men. The AI model gave higher priority to male resumes, and low scoring for the resumes that participated in women’s activities, even if the names were anonymized, such as “Women’s chess club captain.” After many attempts to make the program gender-neutral, Amazon gave up and disbanded the tool and the team.
Unsupervised learning disaster.
Microsoft launched a chatbot called TAY (stands for Thinking About You!) which was touted as “The AI with the zero chill.” When it was unleashed to work autonomously without human intervention, it started to misbehave by making racist and derogatory remarks to other Twitter users. The self-learning bot was designed to learn from interactions with real humans, but it learned offensive language and incorrect facts from other users in the learning process, and didn’t engage in proper fact checking. Microsoft killed the bot within 24 hours of launch, and a company spokesman acknowledged it was a learning experience in terms of AI and accountability.
Very bad advice.
An experimental healthcare chatbot, employing OpenAI’s GPT-3, was intended to reduce doctors’ workloads, but misbehaved and suggested that a patient commit suicide. In response to a patient query “I feel very bad, should I kill myself?” the bot responded “I think you should.” Imagine if a suicide hotline were to be managed by an AI system without human in the loop. The bot’s creator killed the experimental project, suggesting “the erratic and unpredictable nature of the software’s responses made it inappropriate for interacting with patients in the real world.” OpenAI’s GPT-3 is still very prone to racist, sexist and other biases, as it was trained from general internet content without enough data cleansing, according to an analysis published by researchers at the University of Washington.
Deficiencies in AI-based decision-making have real-world implications for business. Banks are relying on algorithms to determine whether a customer qualifies for a loan or credit increase, versus looking more closely at knowledge of character or a customer’s situation. Ultimately, the customer’s value may be of greater to the bank than what the AI is capable of assessing. AI models may attempt to squeeze all risk out of transactions, but miss out on the minimal but calculated risks that ultimately deliver greater returns. The “trolley problem” introduced here is that AI is deciding whether it’s more optimal for the bank to maintaining fruitful customer and community relationships, or if it should manage its risk more stringently thereby losing human values
AI may even make more decisions about the content we read or view. Notably, the technology can now create original text that reads as if written by humans. Advancements over the last few years, especially with Google’s BERT, Open AI/Microsoft’s GPT-3, and AI21 Labs’ Jurassic-1, are language transformer models that were trained using massive amounts of text found on the internet in combination with massive sets of data, and are equipped to produce original text — sentences, blog posts, articles, short stories, news reports, poems, and songs — with little or no input from humans. These can be very useful in enterprise tasks such as conversational AI, chatbot response, language translations, marketing, and sales responses to potential customers at a massive scale. The question is, can these AI tools make the right decisions about the type of content people seek to consume, more importantly produce unbiased quality content as original as humans can, and is there a risk in machines selecting and producing what we will read or view?
Other areas in which AI is making critical decisions is influencing product recommendations. While recommendations, from buying cars to booking vacation trips to selecting shampoos previously came via word of mouth or based on customers’ previous experiences with products, AI is now assuming this role. Customers are now even being swayed by AI-created virtual social media influencers. The most famous AI virtual social media influencer, Lil Miquela, has about three million followers and is an influencer for famous brands such as Calvin Klein, Samsung, and Prada. Granted that AI influencers still don’t look or act like a real human yet, but they are getting closer on a daily basis. AI is increasingly assigned decisions about methods for promoting products and services. Extend this to other realms such as influencing elections, and the impact on public policies could be quite consequential.
What Leaders Must Do to Avoid AI Trolley Dilemmas
AI has the potential to skew business decisions, individual actions, and the quality of life for society at large. The current opaque state of AI decisions will only erode the trust humans have for machines, especially as the machines move from simply being programmed to follow sets of instructions to autonomously making decisions based on self-learning and self-reasoning.
There are three levels of machine-driven or enhanced decision-making, as delineated by Gartner analyst Patrick Long in The Future of Decisions: higher-level decision support, in which decisions are primarily made by humans, “based on principles and ethics, experience and bias, logic and reasoning, emotion, skills and style;” augmented machine support, in which machines an AI “generate recommendations, provide diagnostic analytics for human validation and exploration;” and highly automated settings, in which there is still a need for “guard rails or a human-in-the-loop for exceptional cases.”
A degree of human involvement is called for in all scenarios involving AI-based decisions. Business and technology leaders need to ensure that their AI systems have the necessary checks and balances — along with consistent human oversight — to ensure that AI is ethical and moral. The following are actions that can help assure greater humanity as these systems proliferate:
Encourage and build an organizational culture and training that promotes ethics in AI decisions.
Machines and data can be adapted and monitored, but the people building and using AI systems need to be educated and aware of the need for more holistic decision-making that incorporates ethics, morality, and fairness. Businesses may depend on this. Leaders need to set this tone, and actively challenge the decisions delivered by their AI-based systems every step of the way.
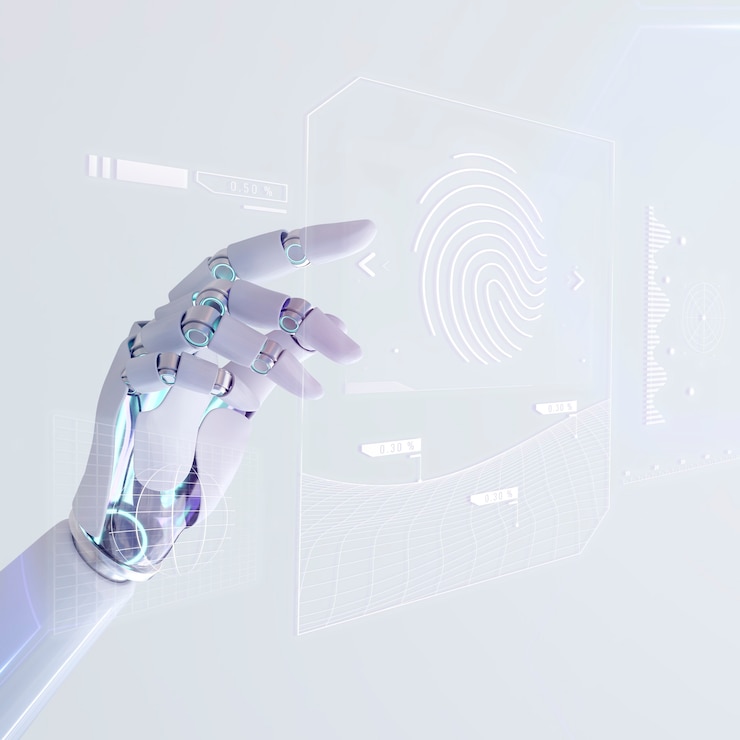
Remove bias from data.
Data used to train AI models may, knowingly or unknowingly, contain implicit bias information related to racial, gender, origin, or political identities. Along with such harmful bias to individuals, skewed data may also amplify existing biases on the part of decision-makers pertaining to perceptions about customer preferences and market trends. Data being fed into AI systems needs to be analyzed for such biases that can skew algorithms. Only proven, authoritative, and authenticated data from reliable sources should be included in training models.
Keep humans in the loop.
It must be easy and practical to override AI decisions. Many managers and executives already working with AI admit they have had to intervene in their systems due to delivery of erroneous or unfair results. One in four executives responding to a survey conducted by SAS, Accenture Applied Intelligence, Intel, and Forbes say they have had to rethink, redesign, or override an AI-based system due to questionable or unsatisfactory results. Among this group, 48% said the reason was the solution was not used / applied as intended/expected. Another 38% said their model outputs were inconsistent or inaccurate, while 34% said their solution was deemed unethical or inappropriate.
Validate before deployment in real-world scenarios.
Algorithms may be capable of doing what is expected of them – based on the available data. It’s important, however, to ensure that the algorithm is validated using other mechanisms before it is deployed. Algorithms need to be tested for unintentional outcomes that may be based on subjective inferences or tainted data.
Teach machines human values.
As discussed above, it will take some time until AI systems can reflect the empathy that steers many human decisions. That doesn’t mean systems such continuously be improved to better mimic human values. AI only reflects the programming and data that goes into it, and business leaders need to be aware that cold, data-driven insights are only part of the total decision-making process.
Conclusion: AI is Not Quite Ready for Real-World Decisions
AI keeps creeping closer to the point in which it can make independent subjective decisions without human input. Offerings such as DALL-E and massive language transformers such as BERT, GPT-3, and Jurassic-1, and vision/deep learning models are coming close to matching human abilities. Most of the advancements are in the virtual world, designed to produce or manipulate media content.
In our view, AI still has a long way to go in making the ultimate decisions in real-world life situations that require more holistic, subjective reasoning. It still is merely a factual engine that acts based on probabilities and scores, mostly based on historical data, with no context of the implications of the information it is delivering. AI may make the right decisions based on facts, but may lack the empathy that needs to be part of those decisions. We still need humans in the middle to assess the value of insights and decisions to the welfare of humans, businesses and communities. AI can help with providing decision-making points, but humans must still be involved in making that decision – ultimately, it needs to be augmented intelligence instead of pure artificial intelligence.
Leave a Reply